I have written extensively about the blittable format already, so I’ll not get into that again. But what I wanted to do in this post is to discuss the implication of the intersection of two very important features:
- The blittable format requires no further action to be useful.
- Voron is based on a memory mapped file concept.
Those two, brought together, are quite interesting.
To see why, let us consider the current state of affairs. In RavenDB 3.0, we store the data as json directly. Whenever we need to read a document, we need to load the document from disk, parse the json, load it into .NET objects, and only then do something with it. When we just got started with RavenDB, it didn’t actually matter to us. Our main concern was I/O, and that dominated all our costs. We spent multiple releases improving on that, and the solution was the prefetcher.
- Prefetcher will load documents from the disk and make them ready to be indexed.
- The prefetcher is running concurrently to indexing, so we can parallelize I/O and CPU work.
That allow us to reduce most of the I/O wait times, but it still left us with problems. If two indexes are working, and they each use their own prefetcher, then we have double the I/O cost, double the parsing cost, double the memory cost, double the GC cost. So in order to avoid that, we group indexes together that are roughly at the same space in their indexing. But that lead to a different set of problems, if we have one slow index, that would impact all the other indexes, so we need to have a way to “abandon” an index while it is indexing, to let the other indexes in the group the chance to run.
There is also another issue, when inserting documents into the database, we want to index them, but it seems stupid to take the index, write it to the disk, only to then load them from the disk, parse them, etc. So when we insert a new document, we add it to the prefetcher directly, saving us some work in the common case where indexes are caught up and only need to index new things. That, too, have a cost, it means that the lifetime of such objects tend to be much longer, which means that they are more likely to be pushed into Gen1 or Gen2, so they will not be collected for a while, and when they do, it will be a more expensive collection run.
Oh, and to top it off, all of the structure above need to consider available memory, load on the server, time for indexing batch, I/O rates, liveliness and probably a dozen other factors that don’t pop to mind right now. In short, this is complex.
With RavenDB 4.0, we set out to remove all of this complexity. A large part of the motivation for the blittable format and using Voron are driven by the reasoning below.
If we can get to a point where we can just access the values, and reading documents won’t incur a heavy penalty in CPU/memory, we could radically shift the cost structure. Let us see how. Now, the only cost for indexing is going to be pure I/O, paging the documents to memory when we access them. Actually indexing them is done by merely access the mapped memory directly, so we don’t actually need to allocate much memory during indexing.
Optimizing the actual I/O is pretty easily done by just asking the operating system, we can do that explicitly using PrefetchVirtualMemory or madvise(MADV_WILLNEED), or just let the OS handle that based on actual access pattern. So those are two separate issues that just went away completely. And without needing to spread the cost of loading the documents among all indexes, we no longer have a good reason to go with grouping indexes. So that is out the window, as well as all the complexity that is required to handle a slow index slowing down everyone.
And because newly written documents are likely to be memory resident (they have just been accessed, after all), we can just skip the whole “let us remember recently written documents for the indexes”, because by the time we index them, we are expecting them to still be in memory.
What is interesting here is that by using the right infrastructure we have been able to remove quite a lot of code. Now, the major part here is that being able to remove a lot of code is almost always great, the major change here is that all of the code we removed had to deal with a very large number of factors (if new documents are coming in, but indexing isn’t caught up to them, we need to stop putting the new documents into the perfetcher cache and clear it) that are hard to predict and sometimes interact in funny ways. By moving a lot of that complexity to “let us manage what parts of the file are memory resident”, we can simplify a lot of that complexity and even push much of it directly to the operation system.
This has other implications, because we now no longer need to run indexes in groups, and they can each run and do their own thing, we can now split them so each index has their own dedicated thread. Which mean, in turn, that if we have a very busy index, it is going to be very easy to point which one is the culprit. It also make it much easier for us to handle priorities. Because each index is a thread, it means that we can now rely on the OS prioritization. If you have an index that you really care about running as soon as possible, we can bump its priority higher. And by default, we can very easily mark the indexing thread as lower priority, so we can prioritize answer incoming requests over processing indexes.
Doing it in this manner means that we are able to ask the OS to handle the problem of starvation in the system, where an index doesn’t get to run because it has a lower priority. All of that is already handled in the OS scheduler, so we can lean on that.
Probably the hardest part in the design of RavenDB 4.0 is that we are thinking very hard about how to achieve our goals (and in many cases exceed them) not by writing code, but by not writing code. But by arranging things so the right thing would happen. Architecture and optimization by omission, so to speak.
As a reminder, we have the RavenDB Conference in Texas in a few months, which would be an excellent opportunity to learn about RavenDB 4.0 and the direction in which we are going.
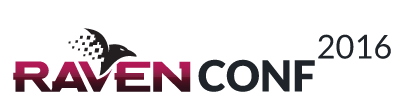